A Jug of Gin, A Leg of Racehorse—and Thou
Recent Cambridge University research, published in the journal Philosophy and Technology, warns about the dangers of machine learning. The below is taken from the Daily Telegraph.
Artificially intelligent recruitment programmes are discriminating against people who wear glasses or sit in front of bare walls, academics have warned, as they urged companies to stop relying on pseudoscientific software.
In video interviews, AI programmes tended to favour people sitting in front of bookshelves or with art on their walls. They also recommended applicants wearing head scarfs, believing them less neurotic, while judging people who wore glasses as less conscientious.
The team said using AI to judge personality was automated pseudoscience reminiscent of physiognomy or phrenology- the discredited beliefs that character can be deduced from facial features and skull shape.
We are concerned that some vendors are wrapping snake oil products in a shiny package and selling them to unsuspecting customers, said co-author Dr Eleanor Drage.
Pseudoscientific?! Someone once said to treat every obstacle as an opportunity. So here goes.
Probability, statistics, risk and return? Yawn! Artificial intelligence? LLM, self-organizing maps,…Yeah, baby!
IBM, M&S, Walmart stocks? Booooring! Alternative investments? Crypto, wine,…Coooooool!
Scenario: You have ambitions to be a hedge fund manager. But the competition for investment money is stiff. You might have some programming skills and a LinkedIn profile. What more do you need? You need a gimmick, to make you stand out from the riff-raff.
Solution: Artificial intelligence for alternative investments…AI for AI…it would be a crime not to! That’s our gimmick. Let’s do the math.
Here are the sectors for the (boring) classical investments: Energy, Real Estate, Financials, IT, Materials, Healthcare, Industrials, Consumer Staples, Utilities, Consumer Discretionary, Communication Services.
Let’s get creative with some less, ahem, traditional investments. I’m going to include a few fairly standard investments, but then I’m going to let rip. In increasing order of weirdness: Sterling, Gold, Oil, Coffee, Bitcoin, UK houses, Wine, Racehorses, Diamonds, Online advertising, Rolex Submariner watches, Gin, Chocolate bars, Crime in Chicago.
No, your eyes aren’t playing tricks on you, I’ve included gin, chocolate, diamonds. And crime. Does it pay? Let’s see.
I downloaded data for all of the classical sectors and the alternatives. Getting data for the alternatives was tricky to say the least. But, hey, that’s not an obstacle, it’s an opportunity! No other potential hedge fund manager is going to be looking at the same data as us!
For example, for racehorses I got data from Statista for the sale prices of racehorses in Ireland. I
know from personal experience that the sale price of a racehorse does not capture the realities of investing in them. The costs of ownership are staggering. But, hey ho. Data for Rolex Submariner watches from Googletrends. Simply interest shown in this particular watch. Not in the slightest bit financial. Crime in Chicago, ok, I couldn’t resist. Is there still any link between crime in Chicago and the liquor trade, or was that link broken almost a century ago? Data from the City of Chicago website. The data is not financial, simply quantity of crimes each year. No allowance for quality.
I converted all data into US dollars, that was more art than science. And looked at annual returns over ten years. I then applied a wonderful machine-learning method called self-organizing maps (SOM).
SOM is an unsupervised-learning method that groups together data points using distance between the feature vectors (here the returns). The data points are then mapped onto a two-dimensional grid, think chessboard, so we can visualize which data points/feature vectors have similar characteristics. Or think moving similar people to the same table at a wedding. And the closer the tables the more similar the people at those tables will be. Tables far apart are very dissimilar, the aunts and uncles no one talks about, or to. (One way that the wedding-table analogy falls down is that at weddings there are usually the same number of people at each table. In SOM some tables are more popular than others.)
And this is what I found.
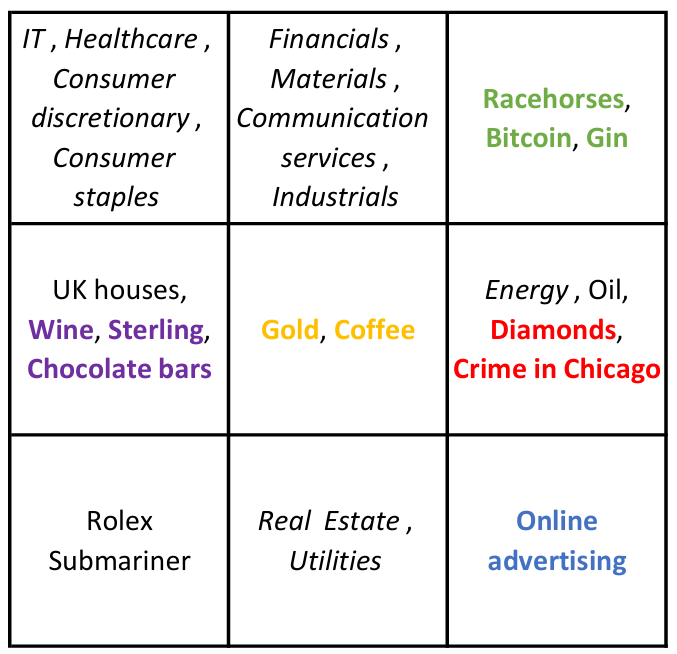
Each cell represents ‘investments’ that are similar. So IT, Healthcare, etc. are similar to each other and very different to racehorses and gin. No kidding. By spreading money across the nine categories we increase diversification and so reduce risk. So some IT shares, a financial or two, a racehorse, definitely plenty of gin, some diamonds (maybe pass on the crime), and so on.
This is then the information that goes into our pitch for raising money for our hedge fund. “Our fund is unique in applying cutting-edge artificial intelligence to portfolios of alternative investments. By exploiting the non-linear blah blah maximization blah blah we construct portfolios to optimize diversity and so blah blah.” Irresistable to those allocating money to new funds. (And if we get comped watches, fine wines, diamonds, and a racehorse leg or two, well, so be it.)
And the point of this exercise?
The beauty of machine learning is that it is fun to do. Usually very easy, there is always some Python code you can use. The hardest part of putting together a pitch like this is in finding the data in the first place, and choosing the best typeface for the pitchbook.
You also always get an answer. With classical mathematical modelling, you might spend months or years perfecting your model, only to find a flaw and have to throw it all away. But not having a clue what the algorithm is doing inside its black box means you might not find out that there is a major problem until after you’ve lost all of your investors’ money.
But not knowing what is inside the box is not a bad thing. If you can’t see it, then nor can your investors. Remember, these aren’t obstacles, they are opportunities.
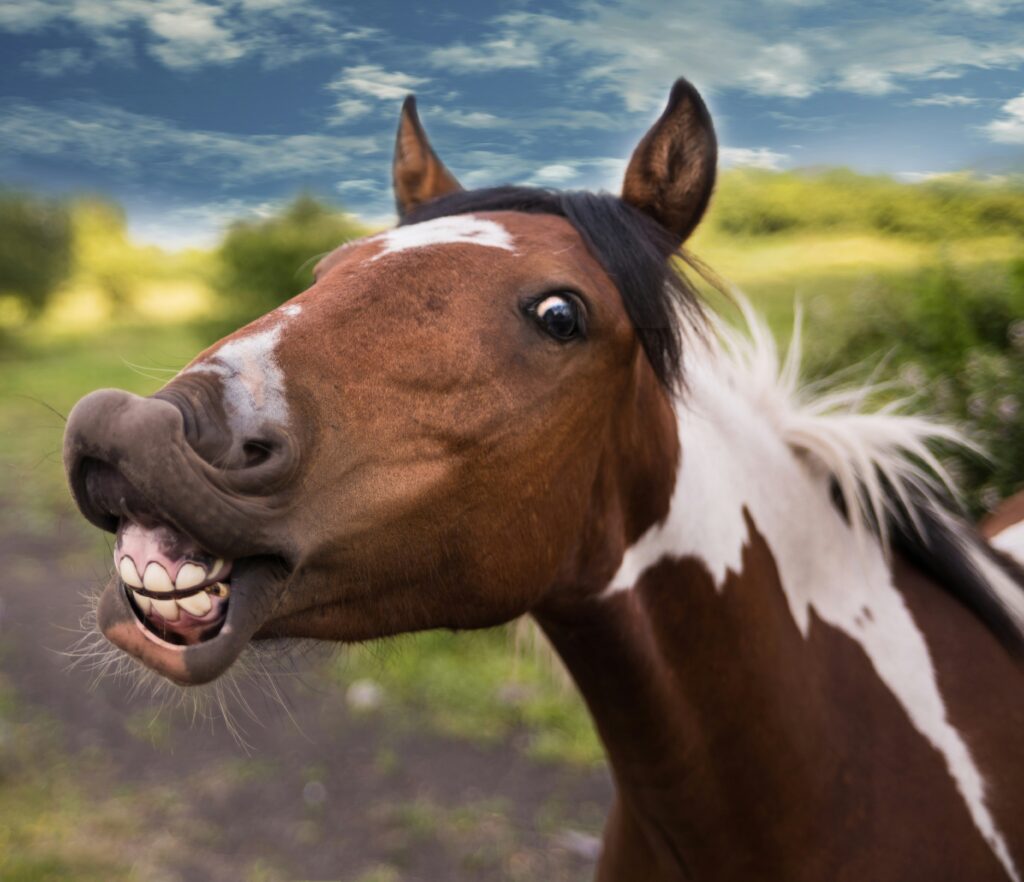
Disclaimer: This information is for general informational purposes only and does not constitute financial, legal, or tax advice. It is not intended to be a substitute for professional advice. You should consult with a qualified professional before making any financial decisions. Past performance is not indicative of future results. The information provided is not an offer to sell or a solicitation of an offer to buy any securities or other financial instruments. We are not responsible for any losses or damages that may arise from your reliance on this information. I hope all that is obvious.
Photo by Magdalena Smolnicka on Unsplash